The Ultimate Guide to Image Data Annotation
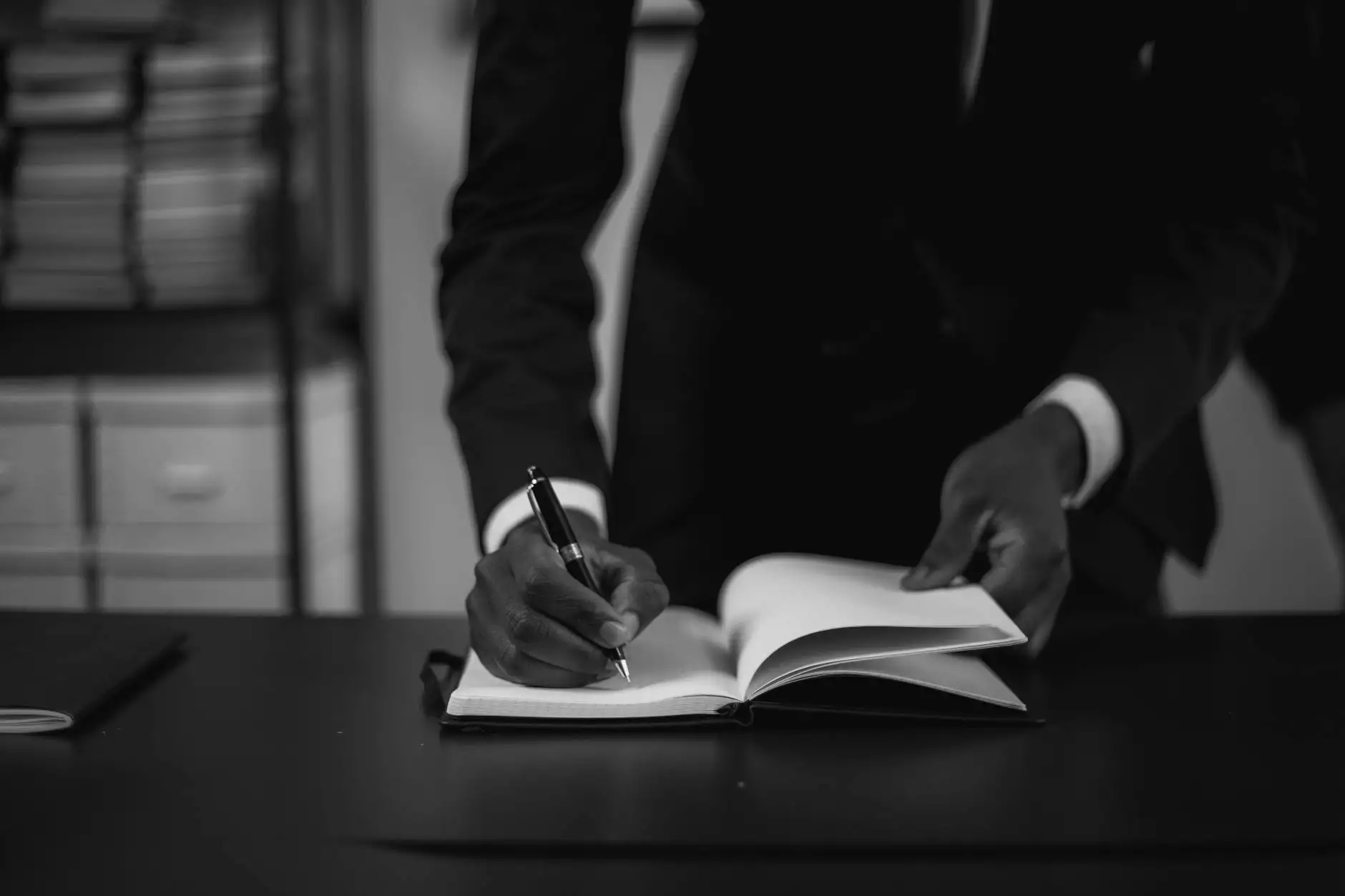
In today's data-driven world, the need for accurate and high-quality datasets is more crucial than ever. Specifically, the realm of image data annotation has gained unparalleled importance with the rise of artificial intelligence (AI) and machine learning (ML) applications. This article delves deep into the significance, tools, platforms, and best practices surrounding image data annotation, aimed at reinforcing the foundational layers of your AI models and solutions.
Understanding Image Data Annotation
Image data annotation is the process of labeling images for the purpose of training machine learning models. This method provides the necessary context to algorithms, enabling them to comprehend visual data in a manner akin to humans. Through precise annotation, AI systems can identify objects, recognize scenes, and interpret complex images, allowing for applications across various industries.
The Importance of Image Data Annotation
Annotation plays a pivotal role in the success of machine learning projects. Without properly annotated data, the effectiveness of models can greatly diminish. Here are several reasons why image data annotation is essential:
- Enhances Model Accuracy: Accurate annotation improves the predictive capabilities of models, leading to better performance in real-world applications.
- Facilitates Object Recognition: Annotated images train models to recognize a wide variety of objects, enabling the development of applications like self-driving cars and facial recognition software.
- Streamlines Data Processing: Annotations make it easier to sort and manage large datasets, which is crucial for scaling AI solutions.
- Supports Diverse Applications: From healthcare imaging analysis to agricultural monitoring, image data annotation supports a myriad of applications across different sectors.
Types of Image Data Annotation
Various methods of image data annotation exist, each tailored to specific needs. Understanding the types allows businesses to choose the right approach:
1. Bounding Box Annotation
This method involves drawing a box around a specific object within an image. It’s particularly useful in scenarios like object detection, where the model learns to locate and categorize various items.
2. Semantic Segmentation
In semantic segmentation, every pixel in the image is classified into a category. This approach is vital for applications requiring detailed understanding, such as autonomous driving and image editing.
3. Instance Segmentation
A more advanced version of semantic segmentation, instance segmentation, differentiates between separate objects of the same class. This allows for a more granulated understanding of the image, facilitating complex tasks in AI.
4. Landmark Annotation
Common in facial recognition technologies, landmark annotation identifies and labels specific points (like eyes, nose, or mouth) on an object. This method is essential for applications focusing on human features.
Choosing the Right Data Annotation Tool
Selecting an appropriate data annotation tool is critical for ensuring efficient and accurate image data annotation. A robust tool should include features such as:
- User-Friendly Interface: The tool should be easy to navigate, allowing annotators to work efficiently.
- Collaboration Features: Enables multiple team members to work together, enhancing productivity and consistency.
- Integration Capabilities: Should seamlessly integrate with existing AI platforms and workflows.
- Quality Assurance Mechanisms: Implement systems to ensure the accuracy and quality of annotations, which is vital for model training.
Exploring Data Annotation Platforms
Companies like KeyLabs.ai provide comprehensive data annotation platforms that cater to diverse industries needing top-tier image annotation services. Here’s a closer look at what makes these platforms essential:
1. Scalable Solutions
Scalable solutions are crucial as businesses grow. Effective data annotation platforms can handle thousands of images simultaneously, ensuring that project timelines are met without compromising quality.
2. Customizable Annotation Options
Different industries have varied requirements for annotation. A versatile platform provides customizable tools that can adapt to specific needs, whether it's facial recognition for security applications or detailed medical imaging.
3. Cost-Effectiveness
Many organizations seek cost-effective solutions without sacrificing quality. The right data annotation platform offers competitive pricing while maintaining high standards.
4. Support and Training
Comprehensive support and training resources are essential for overcoming challenges that may arise during the annotation process. Users should have access to training materials and responsive customer support.
Best Practices for Image Data Annotation
To maximize the effectiveness of image data annotation, businesses should consider several best practices:
- Define Clear Guidelines: Establishing clear annotation guidelines helps ensure consistency and accuracy across the team.
- Utilize Multiple Annotators: Having multiple people annotate the same set of images can balance individual biases and improve overall quality.
- Regular Quality Checks: Implement ongoing quality assurance checks to monitor the accuracy of annotations. These evaluations help in identifying areas for improvement.
- Invest in Training: Well-trained annotators produce better results. Investing in continuous training for the annotation team enhances skills and boosts efficiency.
The Future of Image Data Annotation
As technology evolves, so too will the methods and tools for image data annotation. The integration of artificial intelligence in the annotation process itself is burgeoning, leading to more automated solutions that can increase speed and efficiency while reducing human error.
Innovations in machine learning and computer vision will drive the emergence of next-generation data annotation tools that learn from previous annotation efforts, thereby further streamlining the entire process. These advancements will not only reduce the time required for image annotation but also enhance model outcomes significantly.
Conclusion
In conclusion, the importance of image data annotation cannot be overstated. As AI technology and machine learning models continue to pervade every aspect of business and everyday life, the need for annotated data will only grow. By choosing the right tools and adhering to best practices, organizations can ensure that their machine learning projects stand a good chance of success. Embracing robust annotation systems, such as what KeyLabs.ai offers, empowers businesses to harness the incredible potential of AI and machine learning effectively.