Unlocking the Power of Bounding Boxes in Data Annotation
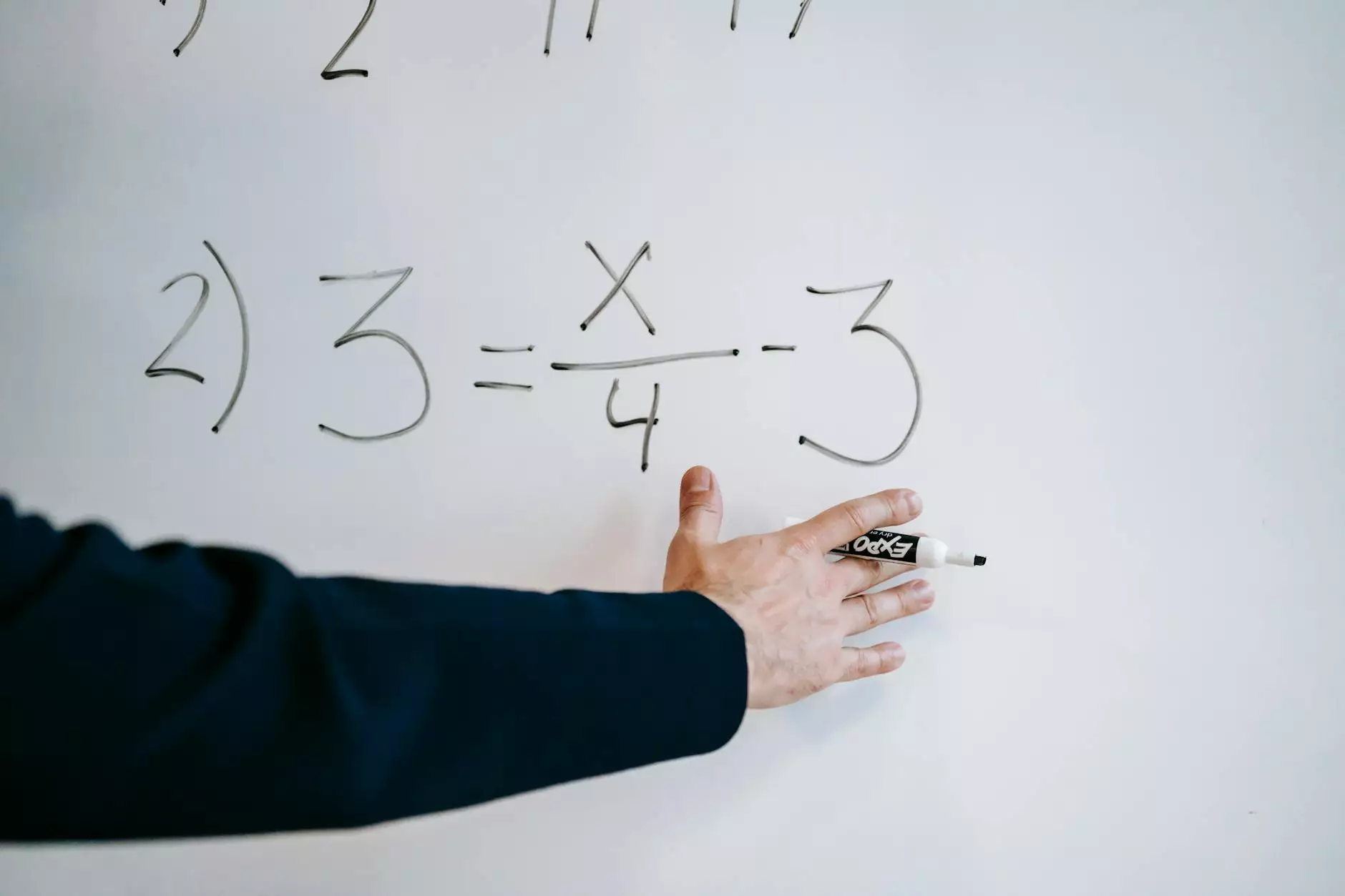
In today's technology-driven world, the term bounding boxes plays a crucial role across myriad applications in computer vision and machine learning. As businesses increasingly rely on advanced imagery data to enhance their operational capabilities, understanding the fundamental concepts behind bounding boxes becomes imperative. This article will delve into the significance of bounding boxes, their applications, and best practices suited for data annotation platforms, particularly through the lens of Keylabs.
The Concept of Bounding Boxes
At its core, a bounding box is a rectangular box that encompasses objects within an image. This powerful tool facilitates the effective identification and localization of various elements in a digital format. Specifically, it provides a convenient method for annotating images, especially when working with machine learning and artificial intelligence (AI) models.
Understanding the Structure of Bounding Boxes
- Coordinates: A bounding box is typically defined by its top-left corner (x, y) coordinates and its width and height.
- Dimensions: The box's dimensions describe the extent of the area surrounding the selected object, crucial for training machine learning models.
- Object Identification: Each bounding box can be tagged with labels that specify the nature of the objects it surrounds (e.g., cars, pedestrians, etc.).
The Importance of Bounding Boxes in Data Annotation
When discussing data annotation tools and platforms, bounding boxes are unavoidable elements that streamline the training of computer vision algorithms. Let’s explore why they are indispensable:
1. Enhanced Object Detection
The primary function of bounding boxes is to enhance object detection. By providing clear demarcation of an object within an image, machine learning models can efficiently learn and identify specific patterns associated with different objects. For example, in self-driving technology, bounding boxes help identify pedestrians, bicycles, and other vehicles on the road.
2. Accuracy in Image Processing
Bounding boxes contribute to increased accuracy in image processing tasks. When models can precisely localize an object, they can better understand its properties and behaviors, leading to more robust predictions. This is particularly crucial in fields such as medical imaging, where accurate object localization (e.g., tumors in scans) can significantly impact treatment planning.
3. Versatility Across Domains
Bounding boxes are versatile and can be applied in various domains, including:
- Retail: Analyzing customer behavior through video feeds to understand shopping patterns.
- Healthcare: Enhancing diagnostic tools by identifying anomalies in medical imagery.
- Manufacturing: Implementing quality control by recognizing defects in production lines.
- Security: Monitoring and identifying potential threats through surveillance cameras.
Bounding Boxes in the Realm of AI
Bounding boxes are a crucial component of AI training datasets, especially for tasks involving computer vision. Their effectiveness lays the foundation for several advanced applications.
1. Image Recognition Models
In machine learning, image recognition models are trained using datasets composed of annotated images with bounding boxes. These models learn to recognize numerous objects by adjusting their parameters based on the bounding box data. A well-annotated dataset, featuring accurate bounding boxes, significantly increases the model's performance and reliability.
2. Real-time Object Tracking
Bounding boxes enable real-time object tracking systems, which utilize frame-to-frame continuity. In applications such as autonomous vehicles and drone-based surveillance, bounding boxes allow for the ongoing location updates of tracked objects, ensuring that the system can predict and respond to dynamic environments effectively.
Implementing Bounding Boxes in Data Annotation Strategies
To harness the full potential of bounding boxes in data annotation, businesses should implement strategic frameworks and utilize the right tools.
1. Choosing the Right Data Annotation Tool
Investing in a high-quality data annotation tool is essential. Platforms like Keylabs offer sophisticated features that streamline the creation of bounding boxes and enhance data quality. A tool with integration capabilities and user-friendly interfaces can save time and improve accuracy.
2. Best Practices for Bounding Box Annotation
- Consistency is Key: Maintain uniformity in the dimension and placement of bounding boxes to avoid training discrepancies.
- Minimize Overlapping Boxes: Ensure that each bounding box distinctly encapsulates a single object to promote clear identification.
- Quality Control: Implement regular audits and quality checks to maintain the integrity and precision of the annotation process.
- Use Expert Annotators: Employ skilled evaluators who can accurately identify and annotate various objects for complex datasets.
Future Trends and Innovations
As the fields of computer vision and AI evolve, the implementation of bounding boxes will continue to transform. Innovations in deep learning and the advent of new technologies like edge computing promise to enhance the capabilities and applications of bounding boxes considerably.
1. Automation in Annotation
Future data annotation platforms will likely leverage AI to automate the creation of bounding boxes, therefore enhancing efficiency. Automated solutions can improve productivity significantly and reduce human error, allowing for rapid scaling of annotation processes.
2. Advanced Annotation Techniques
Emerging methodologies, such as polygonal annotations and segmentation, will coexist with bounding boxes to provide more refined results. These techniques will enable more intricate object representations, beneficial in complex scenarios, such as landscape imagery where simple rectangles may not suffice.
Conclusion
Bounding boxes are vital components of data annotation tools and platforms that underpin many AI-powered applications today. Businesses that harness this technology, and understand the nuances of its implementation, will gain a competitive advantage in their respective industries. At Keylabs, we are dedicated to optimizing data annotation processes to ensure high standards of accuracy and efficiency while embracing the evolving landscape of emerging technologies. By prioritizing bounding boxes in our data annotation strategy, we are setting the stage for future innovations that will shape the technological world.